Why JuiceFS Is Ideal for Hybrid Cloud and Multi-Cloud Environments?
Unified data access across clouds
Cloud-agnostic design
Automated cross-region data distribution
Scenario 1: Cross-cloud and cross-region data distribution for large-scale AI applications
- Synchronizes metadata and data across regions, allowing remote clusters to achieve local-level latency and IOPS.
- Prioritizes access to cached data on local clouds, reducing latency, bandwidth consumption, and costs while improving system performance and stability.
- Automatically synchronizes data across multiple regions, significantly reducing the cost of managing multi-cloud data.
- Real-time monitoring of metadata synchronization ensures data stability and consistency.
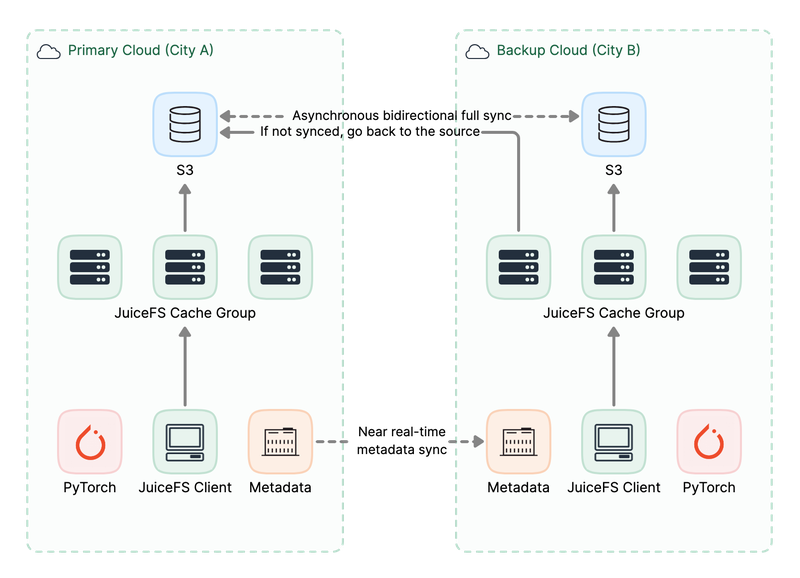
Option 1: Full synchronization of object storage for better performance
- Data and metadata are accessed locally, resulting in better data access performance.
Option 2: On-demand synchronization of object storage for cost efficiency
- Only a distributed cache is used on the remote cloud, and data is preloaded on demand. This avoids bucket replication to significantly reduce storage costs.
- You can use idle NVMe SSDs on GPU clusters to store the cache. This further reduces resource costs.
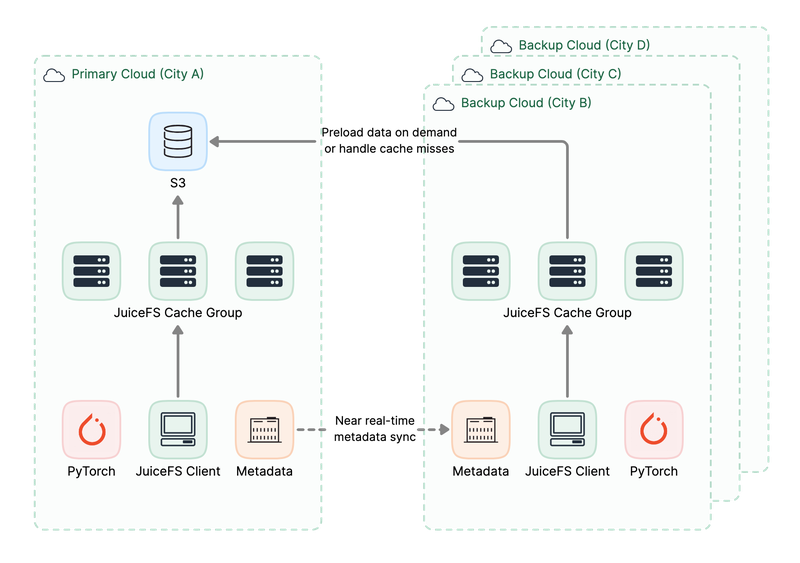
Scenario 2: Same-region, cross-cloud data distribution and disaster recovery
- Independent training on multiple clouds with asynchronous bidirectional synchronization of object storage buckets across clouds, ensuring applications are unaware of the transition.
- Shared metadata service ensures strong metadata consistency.
- Clouds serve as disaster recovery roles for each other. If one cloud's data is inaccessible, you can smoothly switch to another cloud.
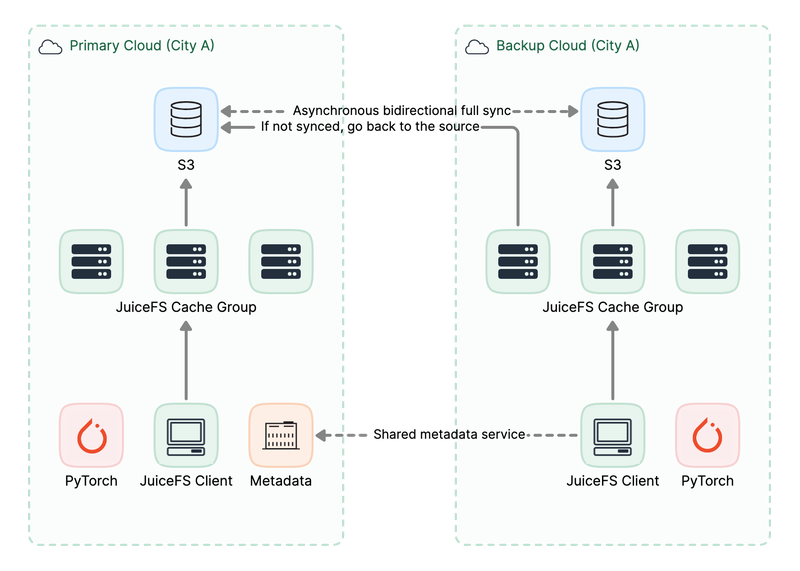
Features
Powering Enterprises in Hybrid and Multi-Cloud Architectures
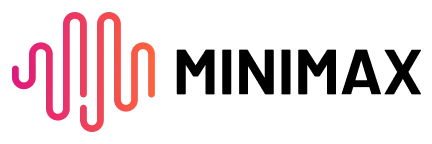

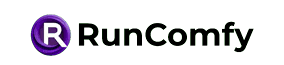
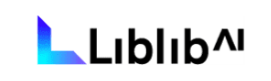